institut
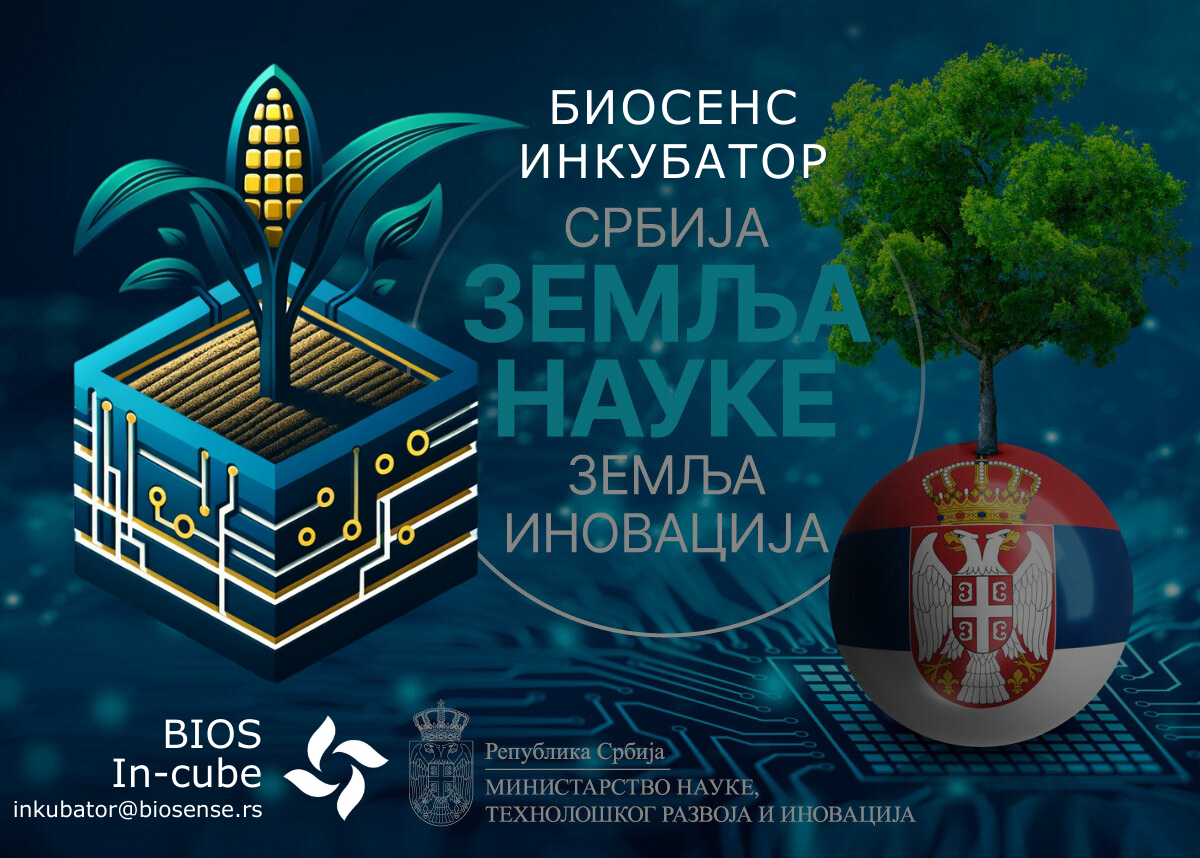
BIOS In-Cube inkubator
Inkubator za sveže ideje
Projekti
Projekat ANTARES - Centar izvrsnosti
ANTARES ima za cilj da pretvori Institut BioSens u Evropski centar izvrsnosti (CoE) za napredne tehnologije u održivoj poljoprivredi, kao i da pruži vrhunska digitalna rešenja...
BioSens knjiga
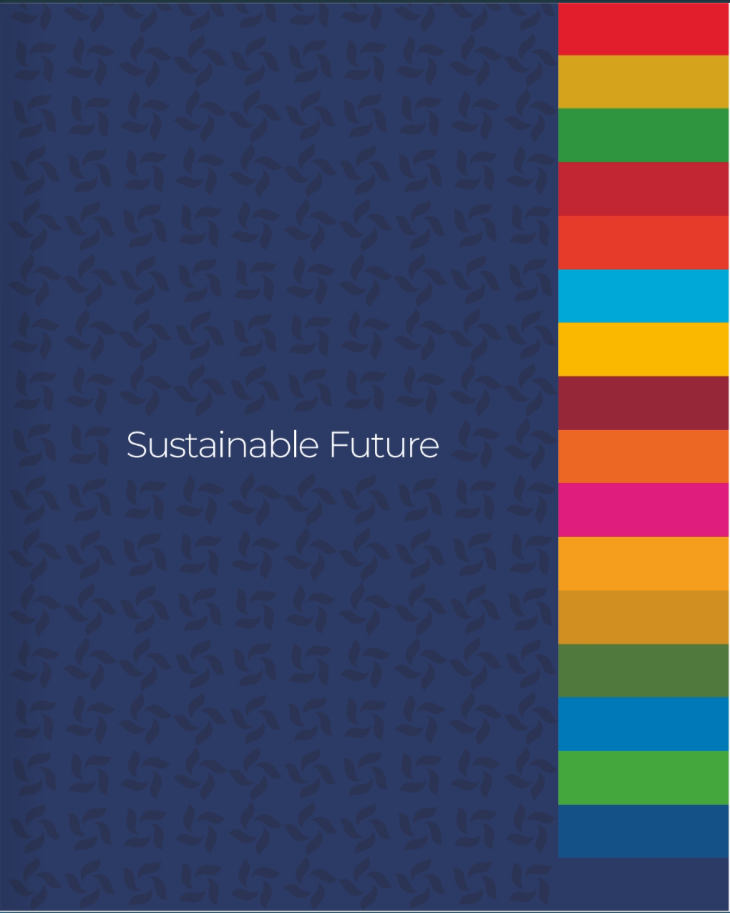
Sustainable Future
Inovacije
AgroSens
AgroSens je digitalna platforma koja pruža podršku poljoprivrednicima i poljoprivrednim kompanijama u praćenju stanja useva i planiranju poljoprivrednih aktivnosti.
Projekti
Advance_eLTER - Advancing the European Long-Term Ecosystem, critical zone and socio-ecological Research Infrastructure towards ESFRI
Cilj projekta: Advance eLTER predstavlja presudan korak u izgradnji evropske istraživačke infrastrukture lokacija za dugoročno istraživanje ekosistema i društveno-ekoloških istraživačkih platformi (eLTER RI), a sve to kako bi pružio najkvalitetnije...
saradnja
BioSens akcelerator
Lansirajte svoju AgTech ideju!
BioSens akcelerator je intenzivan, tromesečni program namenjen AgTech startapima u ranoj fazi i predstavlja snažan most između nauke i biznisa. Program omogučava...
Ljudi

Upoznajte Mirjanu Radulović
Vi radite na Institutu BioSens, ali u isto vreme ste i doktorand. Koliko je važno...
Inovacije
Komunikacije
Istraživanje BioSensovog Centra za senzorske tehnologije je usmereno ka razvoju uređaja koji mere optička, fizička i elektromagnetna svojstva svoje okoline....
saradnja
Digitalni Inovacioni Habovi
BioSens Institut (Istraživačko – razvojni institut za informacione tehnologije biosistema) je vodeći srpski Digitalni Inovacioni...
saradnja
Živa laboratorija
Istraživanja i razvoj inovacija na Institutu BioSens uvek se odvijaju u saradnji sa farmerima, poljoprivrednim i vladinim sektorom, preduzetničkom i poslovnom zajednicom, međunarodnim istraživačkim centrima i građanima. Radimo zajedno u...